Publication Date
2024
Document Type
Book
Description
Understanding streaming sensor signals is critical for in-process monitoring and quality inspection for various industry applications, which allows immediate anomaly detection and diagnosis for potential quality issues. Due to the unavoidable acquisition step, the obtained information is overwhelmingly submerged in high-level noises, which may adversely impact the accuracy and effectiveness of extracting information from monitored sensor signals under the nanoscale. To solve this issue, an active learning strategy for the streaming signal data with the shapely embedded ensembling Gaussian process surrogate allows for the recognition of weak signals. This proposed approach is tested by experimental music timbre recognition problems followed by real-time surface morphology characterizations under nanomachining processes. The results indicate that this proposed approach can effectively capture the underlying spectral patterns of acoustics signals. The presented framework opens up an avenue to allow real-time characterizations and quality inspection for surface characteristics under the nanoscale.
Files
Download Full Text (739 KB)
Recommended Citation
Wang, Xinchen; Alshoul, Mohammad; Deng, Jia; and Wang, Zimo, "A Spectrally Active Learning Gaussian Process Surrogate Model for Weak Signal Processing: An Application for Real-time Surface Characterizations of Nanomachining" (2024). Research Days Posters 2024. 102.
https://orb.binghamton.edu/research_days_posters_2024/102
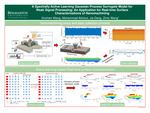